
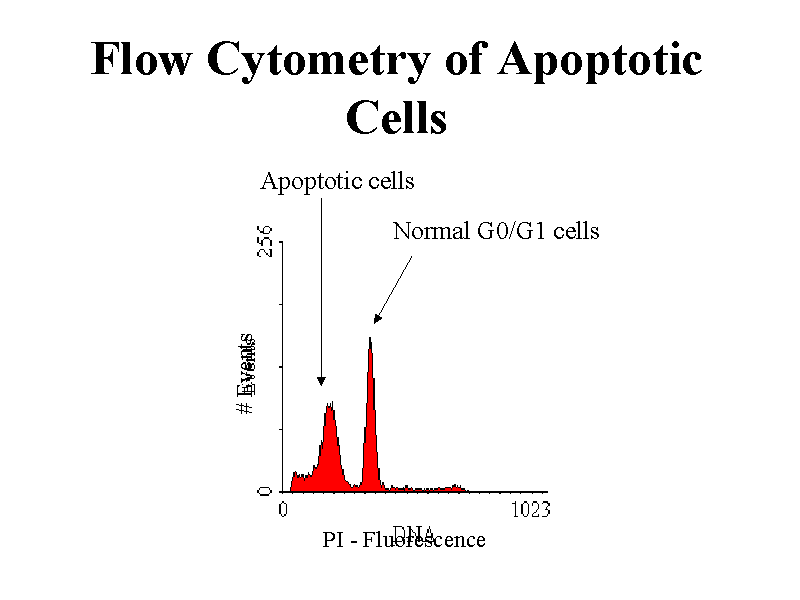
Our overview of the recent publications also highlights that data quality determines analysis quality, but also adds to the challenge of choosing the right model for the data. Of important relevance is the combination of different omics data to identify the contributions of the various regulatory levels. For knowledge-driven analysis, machine learning contributes to biomarker detection, classification and regression, biochemical pathway identification, and carbon flux determination. During processing steps, the supervised machine learning methods help peak picking, normalization, and missing data imputation. We review here commonly used tools, such as random forest, support vector machines, artificial neural networks, and genetic algorithms. In particular, supervised machine learning has great potential in metabolomics research because of the ability to supply quantitative predictions.
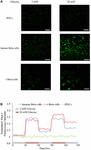
In this review, we address recent developments in using machine learning for processing MS spectra and show how machine learning generates new biological insights. Machine learning methods have become immensely popular for statistical analysis due to the inherent nonlinear data representation and the ability to process large and heterogeneous data rapidly.

However, MS metabolome data analysis is complicated, since metabolites interact nonlinearly, and the data structures themselves are complex. The most popular analytical metabolomics platform is mass spectrometry (MS). Metabolomics helps to understand disease progression in clinical settings or estimate metabolite overproduction for metabolic engineering. Results obtained from its use in a real case study have been analyzed and validated through both literature data and preliminary wet-lab experiments, showing the potential of this tool when performing exploratory analysis.Ĭytoscape Data fusion Network analysis Network modules Pathway analysis Signal transduction pathways microRNA.Abstract: The metabolome of an organism depends on environmental factors and intracellular regulation and provides information about the physiological conditions. To show the potential of the plugin we have applied it in a study of two miRNAs that are particularly relevant in human melanoma progression, miR-146a and miR-214.ĬyTRANSFINDER supports the reconstruction of small signal transduction pathways among groups of genes. Since CyTRANSFINDER has been designed to support exploratory analysis, it does not rely on expression data. We have developed CyTRANSFINDER, a new Cytoscape plugin able to infer three-component signal transduction pathways based on user defined regulatory patterns and including miRNAs, TFs and genes. We observed a lack of computational instruments supporting explorative analysis on this type of three-component signal transduction pathways. However, networks involving a single type of regulator and neglecting transcriptional and post-transcriptional regulations mediated by transcription factors and microRNAs, respectively, may not fully reveal the complex regulatory mechanisms of a cell. Most of the attempts to reconstruct signal transduction pathways are limited to single regulator networks including only genes/proteins. Signal Transduction Pathways are molecular circuits that model how cells receive, process, and respond to information from the environment providing snapshots of the overall cell dynamics. Biological research increasingly relies on network models to study complex phenomena.
